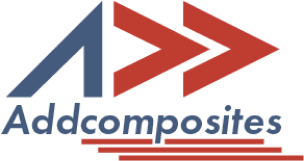
In this comprehensive blog, we delve into the automated fiber placement process, a crucial technique for manufacturing high-performance composite components. We discuss the importance of optimizing process parameters to ensure the highest quality of the final product and examine the factors that affect its quality, including raw material characteristics, mandrel factors, compaction roller dimensions, and heat source variables.
We then explore how Design of Experiments (DOE) can be used to analyze and optimize these parameters, leading to improved voids, crystallinity, and mechanical properties of the composite material. By applying the optimized parameters in production, we can achieve consistent material properties and bond strength, while effectively monitoring and maintaining temperature, pressure, and time.
The blog also covers advancements in process optimization and adaptation, such as the use of digital twin software for continuous product quality monitoring, AI models for data-driven adaptation of process parameters, and the development of more accurate physics-informed AI models to eliminate trial and error.
Lastly, we provide resources for further exploration, including relevant literature, expert consultations, industry forums for knowledge sharing, and training resources for staff involved in the automated fiber placement process.
The manufacturing industry is constantly seeking innovative methods to improve the production of high-quality composite materials. One such technique, which has gained significant traction in recent years, is the Automated Fiber Placement (AFP) process. This process involves utilizing unidirectional carbon fiber-reinforced thermoplastic tapes to create high-performance composite components, which are increasingly finding applications in various industrial sectors. In this blog, we will explore the AFP process and discuss the importance of optimizing the process parameters for manufacturing composite parts.
The Automated Fiber Placement (AFP) process involves using a robotic system to place unidirectional pre-impregnated fiber tapes onto a substrate or mandrel. The tapes are laid along pre-defined paths, allowing for a high degree of freedom in designing the final product. The AFP process is enhanced by incorporating a heat source, which melts the thermoplastic matrix during fiber placement. This enables in situ consolidation of the fiber, eliminating the need for post-processing and curing steps.
The AFP process offers several advantages, including higher productivity, better quality composites, reduced waste material, less labor, shorter production time, improved accuracy and repeatability of the product, and software solutions to streamline the process.
The quality of the final composite part is dependent on various factors, such as void-free and well-consolidated structure, and controlled crystallinity. These factors are influenced by the parameters involved in the AFP process. Therefore, it is crucial to optimize these parameters to achieve the desired outcome.
In this blog, we will discuss some of the key process parameters include: raw material quality, mandrel factors, compaction roller dimensions, heat source variables, and process parameters like heat transfer coefficient, placement rate, and substrate temperature. A thorough understanding and optimization of these parameters can lead to the production of high-quality composite parts that are reliable for use in various structures.
Design of Experiments (DOE) is an approach to systematically design experiments that vary relevant factors to identify optimal conditions. In the context of AFP processes, DOE helps manufacturers analyze and optimize the input parameters to achieve the desired output parameters
Three main output parameters are evaluated after each experiment with DOE: voids, crystallinity, and mechanical properties.
By analyzing the output parameters, manufacturers can determine the ideal combination of input parameters to optimize the AFP process. For instance, it has been observed that the degree of crystallinity and the size of the crystals are higher for samples cooled by higher melting temperatures. Additionally, similar degrees of crystallinity have been obtained in other studies, indicating that PEEK is not sensitive to the cooling rates involved in the AFP process.
In conclusion, implementing optimization techniques like DOE can help manufacturers identify optimal conditions for the AFP process. Analyzing output parameters such as voids, crystallinity, and mechanical properties enables manufacturers to fine-tune their processes to achieve superior product quality and performance.
With the optimal conditions identified through DOE analysis, manufacturers can now proceed to apply these parameters in the production of composite materials using the AFP process. This section will discuss how to adjust the process parameters based on the DOE results, ensure consistency in material properties and bond strength, and monitor and maintain temperature, pressure, and time throughout the process.
The DOE results provide invaluable insights into the ideal combination of input parameters for achieving optimal output parameters. Manufacturers should adjust their process parameters accordingly, ensuring that the fiber placement process is carried out under optimal conditions. Some of the adjustments may include changes to the heat source power, fiber placement speed, compaction roller pressure, and substrate temperature.
Applying the optimized parameters in production is crucial to ensure consistency in material properties and bond strength across the final composite parts. This involves maintaining the ideal degree of crystallinity, minimizing voids, and ensuring strong interlayer bonding. To achieve this, manufacturers should focus on quality control measures throughout the production process. Regular inspection and testing of the produced parts can help identify any deviations from the desired properties and allow for prompt corrective action.
The HAFP/AFP process relies on precise control of temperature, pressure, and time to ensure proper consolidation and bonding of the composite layers. Manufacturers must continuously monitor these parameters during production to maintain consistency and achieve the desired output parameters. Advanced sensors and control systems can be employed to measure and maintain these parameters within the specified range. Any deviations from the set parameters should be promptly addressed to prevent negative impacts on the final product's quality and performance.
As the industry continues to evolve, it is essential to focus on ongoing improvements and adaptation to ensure that the AFP process remains efficient and effective in producing high-quality composite parts. In this section, we will explore the use of digital twin software for continuous monitoring of product quality, building AI models to adapt process parameters for different parts, and developing more accurate physics-informed AI models to minimize the need for trial and error.
Digital twin technology allows manufacturers to create a virtual replica of the production process and monitor the product quality in real-time. By utilizing digital twin software, manufacturers can identify any deviations from the desired parameters and make necessary adjustments to the process. This ensures that the final composite parts meet the required quality standards and reduces the risk of defects or inconsistencies.
Artificial intelligence (AI) models can be employed to analyze the data collected during the AFP process and adapt the process parameters for composite parts of different sizes and shapes. By feeding the AI model with historical data and process parameters, it can identify patterns and trends that may impact the final product's quality. This allows manufacturers to make informed decisions on the process parameters for different parts without relying on trial and error, improving efficiency and reducing waste.
Developing more accurate physics-informed AI models can further enhance the AFP process by minimizing the need for trial and error. These models integrate the underlying physics of the process, such as heat transfer and material properties, with AI-driven algorithms to provide a more accurate representation of the process. By leveraging these models, manufacturers can gain insights into the optimal process parameters for different composite parts and make necessary adjustments without the need for extensive experimentation.
In this comprehensive blog, we delve into the automated fiber placement process, a crucial technique for manufacturing high-performance composite components. We discuss the importance of optimizing process parameters to ensure the highest quality of the final product and examine the factors that affect its quality, including raw material characteristics, mandrel factors, compaction roller dimensions, and heat source variables.
We then explore how Design of Experiments (DOE) can be used to analyze and optimize these parameters, leading to improved voids, crystallinity, and mechanical properties of the composite material. By applying the optimized parameters in production, we can achieve consistent material properties and bond strength, while effectively monitoring and maintaining temperature, pressure, and time.
The blog also covers advancements in process optimization and adaptation, such as the use of digital twin software for continuous product quality monitoring, AI models for data-driven adaptation of process parameters, and the development of more accurate physics-informed AI models to eliminate trial and error.
Lastly, we provide resources for further exploration, including relevant literature, expert consultations, industry forums for knowledge sharing, and training resources for staff involved in the automated fiber placement process.
The manufacturing industry is constantly seeking innovative methods to improve the production of high-quality composite materials. One such technique, which has gained significant traction in recent years, is the Automated Fiber Placement (AFP) process. This process involves utilizing unidirectional carbon fiber-reinforced thermoplastic tapes to create high-performance composite components, which are increasingly finding applications in various industrial sectors. In this blog, we will explore the AFP process and discuss the importance of optimizing the process parameters for manufacturing composite parts.
The Automated Fiber Placement (AFP) process involves using a robotic system to place unidirectional pre-impregnated fiber tapes onto a substrate or mandrel. The tapes are laid along pre-defined paths, allowing for a high degree of freedom in designing the final product. The AFP process is enhanced by incorporating a heat source, which melts the thermoplastic matrix during fiber placement. This enables in situ consolidation of the fiber, eliminating the need for post-processing and curing steps.
The AFP process offers several advantages, including higher productivity, better quality composites, reduced waste material, less labor, shorter production time, improved accuracy and repeatability of the product, and software solutions to streamline the process.
The quality of the final composite part is dependent on various factors, such as void-free and well-consolidated structure, and controlled crystallinity. These factors are influenced by the parameters involved in the AFP process. Therefore, it is crucial to optimize these parameters to achieve the desired outcome.
In this blog, we will discuss some of the key process parameters include: raw material quality, mandrel factors, compaction roller dimensions, heat source variables, and process parameters like heat transfer coefficient, placement rate, and substrate temperature. A thorough understanding and optimization of these parameters can lead to the production of high-quality composite parts that are reliable for use in various structures.
Design of Experiments (DOE) is an approach to systematically design experiments that vary relevant factors to identify optimal conditions. In the context of AFP processes, DOE helps manufacturers analyze and optimize the input parameters to achieve the desired output parameters
Three main output parameters are evaluated after each experiment with DOE: voids, crystallinity, and mechanical properties.
By analyzing the output parameters, manufacturers can determine the ideal combination of input parameters to optimize the AFP process. For instance, it has been observed that the degree of crystallinity and the size of the crystals are higher for samples cooled by higher melting temperatures. Additionally, similar degrees of crystallinity have been obtained in other studies, indicating that PEEK is not sensitive to the cooling rates involved in the AFP process.
In conclusion, implementing optimization techniques like DOE can help manufacturers identify optimal conditions for the AFP process. Analyzing output parameters such as voids, crystallinity, and mechanical properties enables manufacturers to fine-tune their processes to achieve superior product quality and performance.
With the optimal conditions identified through DOE analysis, manufacturers can now proceed to apply these parameters in the production of composite materials using the AFP process. This section will discuss how to adjust the process parameters based on the DOE results, ensure consistency in material properties and bond strength, and monitor and maintain temperature, pressure, and time throughout the process.
The DOE results provide invaluable insights into the ideal combination of input parameters for achieving optimal output parameters. Manufacturers should adjust their process parameters accordingly, ensuring that the fiber placement process is carried out under optimal conditions. Some of the adjustments may include changes to the heat source power, fiber placement speed, compaction roller pressure, and substrate temperature.
Applying the optimized parameters in production is crucial to ensure consistency in material properties and bond strength across the final composite parts. This involves maintaining the ideal degree of crystallinity, minimizing voids, and ensuring strong interlayer bonding. To achieve this, manufacturers should focus on quality control measures throughout the production process. Regular inspection and testing of the produced parts can help identify any deviations from the desired properties and allow for prompt corrective action.
The HAFP/AFP process relies on precise control of temperature, pressure, and time to ensure proper consolidation and bonding of the composite layers. Manufacturers must continuously monitor these parameters during production to maintain consistency and achieve the desired output parameters. Advanced sensors and control systems can be employed to measure and maintain these parameters within the specified range. Any deviations from the set parameters should be promptly addressed to prevent negative impacts on the final product's quality and performance.
As the industry continues to evolve, it is essential to focus on ongoing improvements and adaptation to ensure that the AFP process remains efficient and effective in producing high-quality composite parts. In this section, we will explore the use of digital twin software for continuous monitoring of product quality, building AI models to adapt process parameters for different parts, and developing more accurate physics-informed AI models to minimize the need for trial and error.
Digital twin technology allows manufacturers to create a virtual replica of the production process and monitor the product quality in real-time. By utilizing digital twin software, manufacturers can identify any deviations from the desired parameters and make necessary adjustments to the process. This ensures that the final composite parts meet the required quality standards and reduces the risk of defects or inconsistencies.
Artificial intelligence (AI) models can be employed to analyze the data collected during the AFP process and adapt the process parameters for composite parts of different sizes and shapes. By feeding the AI model with historical data and process parameters, it can identify patterns and trends that may impact the final product's quality. This allows manufacturers to make informed decisions on the process parameters for different parts without relying on trial and error, improving efficiency and reducing waste.
Developing more accurate physics-informed AI models can further enhance the AFP process by minimizing the need for trial and error. These models integrate the underlying physics of the process, such as heat transfer and material properties, with AI-driven algorithms to provide a more accurate representation of the process. By leveraging these models, manufacturers can gain insights into the optimal process parameters for different composite parts and make necessary adjustments without the need for extensive experimentation.