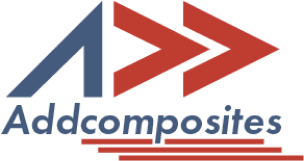
Automated Fiber Placement (AFP) is a critical technology in composite manufacturing, especially for aerospace components. AFP presents significant challenges in data management and analysis due to the complexity of its operations. The challenges arise from handling vast amounts of diverse data generated during various manufacturing stages:
Each stage produces unique data sets that need to be integrated and analyzed to ensure accuracy and quality. The complexity of data integration stems from:
Data mapping is a major challenge in AFP, necessary for aligning and correlating data across different sources and formats. Effective data mapping is crucial for understanding relationships between data points and making informed decisions based on comprehensive analysis. An area not fully explored is the capability for real-time data processing, which is crucial for enabling timely adjustments during manufacturing to correct defects or deviations. Addressing data management challenges through innovative mapping techniques and integrating different data types can significantly optimize AFP operations, leading to reduced waste, increased accuracy, and higher overall efficiency.
Data integration in AFP is particularly challenging due to the multi-modal nature of the data and the high precision required in composite manufacturing. Integrating data from design, process planning, manufacturing, and inspection stages is critical for maintaining accuracy and quality.
An aspect that could be further explored is automated methods for real-time defect detection and correction, which would enhance the efficiency and reliability of AFP operations.
AFP relies heavily on advanced mapping techniques to manage and analyze extensive data collected during complex manufacturing processes. Effective data mapping is crucial for understanding relationships between datasets and improving accuracy and quality of composite manufacturing.
Virtual and physical data avatars simulating the manufacturing environment help in testing and validating data mapping methodologies before application in actual manufacturing scenarios. While the methodologies discussed are comprehensive, they primarily focus on data mapping for defect identification and process optimization.
A possible area not covered extensively is the application of these mapping techniques for predictive maintenance and machine learning models, which could further enhance the predictive capabilities of AFP systems.
Effective data management and advanced mapping techniques in AFP lead to innovative solutions that optimize production and enhance the quality of composite structures.
While these applications of data-driven solutions are groundbreaking, additional potential may not be fully explored:
Scalability and adaptability are crucial for broader application in the industry, ensuring the benefits of these innovations can be realized in various manufacturing environments.
We extend our heartfelt thanks to Alex Brasington, Joshua Halbritter, Matthew Godbold, Max Kirkpatrick, Christopher Sacco, and Ramy Harik from the University of South Carolina, who are the esteemed authors of the influential PDF "Mapping of multimodality data for manufacturing analyses in automated fiber placement." Their groundbreaking work in Automated Fiber Placement (AFP) technology and data mapping methodologies provided the foundational knowledge and insights that greatly enriched this blog.
Their meticulous research and innovative approaches have not only advanced the field of aerospace manufacturing but have also enabled us to share these complex concepts in an accessible format. We are deeply appreciative of their efforts and are privileged to highlight their contributions in our discussions.
Discover the future of composite manufacturing with Addcomposites! Here's how you can get involved:
At Addcomposites, we are dedicated to revolutionizing composite manufacturing. Our AFP systems and comprehensive support services are waiting for you to harness. So, don't wait – get started on your journey to the future of manufacturing today!
Automated Fiber Placement (AFP) is a critical technology in composite manufacturing, especially for aerospace components. AFP presents significant challenges in data management and analysis due to the complexity of its operations. The challenges arise from handling vast amounts of diverse data generated during various manufacturing stages:
Each stage produces unique data sets that need to be integrated and analyzed to ensure accuracy and quality. The complexity of data integration stems from:
Data mapping is a major challenge in AFP, necessary for aligning and correlating data across different sources and formats. Effective data mapping is crucial for understanding relationships between data points and making informed decisions based on comprehensive analysis. An area not fully explored is the capability for real-time data processing, which is crucial for enabling timely adjustments during manufacturing to correct defects or deviations. Addressing data management challenges through innovative mapping techniques and integrating different data types can significantly optimize AFP operations, leading to reduced waste, increased accuracy, and higher overall efficiency.
Data integration in AFP is particularly challenging due to the multi-modal nature of the data and the high precision required in composite manufacturing. Integrating data from design, process planning, manufacturing, and inspection stages is critical for maintaining accuracy and quality.
An aspect that could be further explored is automated methods for real-time defect detection and correction, which would enhance the efficiency and reliability of AFP operations.
AFP relies heavily on advanced mapping techniques to manage and analyze extensive data collected during complex manufacturing processes. Effective data mapping is crucial for understanding relationships between datasets and improving accuracy and quality of composite manufacturing.
Virtual and physical data avatars simulating the manufacturing environment help in testing and validating data mapping methodologies before application in actual manufacturing scenarios. While the methodologies discussed are comprehensive, they primarily focus on data mapping for defect identification and process optimization.
A possible area not covered extensively is the application of these mapping techniques for predictive maintenance and machine learning models, which could further enhance the predictive capabilities of AFP systems.
Effective data management and advanced mapping techniques in AFP lead to innovative solutions that optimize production and enhance the quality of composite structures.
While these applications of data-driven solutions are groundbreaking, additional potential may not be fully explored:
Scalability and adaptability are crucial for broader application in the industry, ensuring the benefits of these innovations can be realized in various manufacturing environments.
We extend our heartfelt thanks to Alex Brasington, Joshua Halbritter, Matthew Godbold, Max Kirkpatrick, Christopher Sacco, and Ramy Harik from the University of South Carolina, who are the esteemed authors of the influential PDF "Mapping of multimodality data for manufacturing analyses in automated fiber placement." Their groundbreaking work in Automated Fiber Placement (AFP) technology and data mapping methodologies provided the foundational knowledge and insights that greatly enriched this blog.
Their meticulous research and innovative approaches have not only advanced the field of aerospace manufacturing but have also enabled us to share these complex concepts in an accessible format. We are deeply appreciative of their efforts and are privileged to highlight their contributions in our discussions.
Discover the future of composite manufacturing with Addcomposites! Here's how you can get involved:
At Addcomposites, we are dedicated to revolutionizing composite manufacturing. Our AFP systems and comprehensive support services are waiting for you to harness. So, don't wait – get started on your journey to the future of manufacturing today!