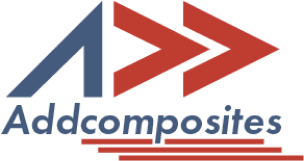
Structural Health Monitoring (SHM) in composite aerostructures presents a complex set of challenges, primarily due to the intricate nature of composite materials and the critical demand for maintaining aerospace safety standards. Composites, characterized by their lightweight and high-strength properties, are increasingly used in aerospace applications, leading to a significant need for effective SHM systems that can detect, localize, and characterize damage accurately.
One of the key challenges in SHM is the detection of damage types that are not readily visible or easily identifiable, such as delaminations or micro-cracks within the composite layers. These damage types can compromise the structural integrity of aerostructures but might not be detectable through traditional inspection methods. Furthermore, the heterogeneous composition of composites adds another layer of complexity, as damage propagation behaves differently compared to homogeneous materials.
The blog highlights the importance of developing sophisticated SHM methodologies capable of overcoming these challenges. It emphasizes the need for systems that can operate under the uncertainties inherent in composite materials, such as variations in material properties, environmental conditions, and operational loads. Achieving this requires a multidisciplinary approach, combining knowledge from material science, mechanical engineering, and data analytics.
Advancements in sensor technologies and machine learning algorithms offer promising solutions to these challenges. By integrating sensors within composite structures, real-time data collection becomes feasible, allowing for the continuous monitoring of structural health. Machine learning algorithms can then analyze this data, learning from patterns and anomalies to detect and characterize damage more accurately.
However, the development and implementation of these advanced SHM systems are not without its hurdles. Technical limitations, such as sensor sensitivity, data processing capabilities, and algorithm robustness, need to be addressed. Additionally, there's a need for extensive validation and testing to ensure reliability and effectiveness in real-world applications.
The task of detecting and characterizing damage within composite aerostructures is notably complicated by the presence of uncertainty. This uncertainty stems from several sources, including the variability in material properties, the influence of environmental conditions on the structure, and the complexities associated with the damage itself, such as its location, size, and type. Traditional methods of structural health monitoring (SHM) often struggle to accurately identify damage under these uncertain conditions, leading to the need for more sophisticated approaches.
Machine learning (ML) offers a powerful solution to this challenge, utilizing algorithms capable of learning from data to detect patterns and anomalies indicative of damage. The application of ML in SHM allows for the analysis of vast amounts of data generated by sensors embedded in the composite structures. These algorithms can adapt to the inherent uncertainties, improving their ability to detect and characterize damage over time.
However, the development and deployment of ML-based SHM systems are not without challenges. The accuracy of these systems heavily depends on the quality and quantity of the data available for training the algorithms. Ensuring that the ML models are trained on comprehensive datasets that accurately represent the range of possible damage scenarios is crucial. Additionally, the interpretability of ML algorithms is essential for gaining the trust of engineers and technicians who rely on these systems to make critical decisions regarding the maintenance and safety of aerostructures.
The advent of machine learning (ML) has significantly shifted the paradigm for damage detection in aerospace composites. By harnessing the power of ML algorithms, the process transcends the limitations of traditional methods, offering a dynamic approach capable of adapting to the complexities inherent in composite materials. This section delves into the methodology and implementation of ML for detecting and characterizing damage within composite aerostructures, highlighting its potential to revolutionize structural health monitoring (SHM).
At the core of this approach is the development of sophisticated ML models trained on data collected from embedded sensors within the composite structures. These sensors provide a continuous stream of data, capturing subtle changes in the material's behavior that may indicate the presence of damage. Through advanced data processing and analysis techniques, ML algorithms can identify patterns and anomalies within this data, flagging potential damage sites for further inspection.
The process involves several key steps, starting with data acquisition and preprocessing to ensure that the ML models receive high-quality inputs. Feature extraction is then performed, isolating the most relevant information from the sensor data to improve the efficiency and accuracy of the detection process. With the features identified, the ML models are trained to recognize the characteristics of damage, learning from examples to refine their predictive capabilities.
Innovating aerospace safety through machine learning (ML) in structural health monitoring (SHM) offers a transformative approach to ensuring the integrity and longevity of composite aerostructures. This section discusses the solution's implementation, emphasizing its potential to revolutionize aerospace safety protocols.
The incorporation of ML into SHM systems enhances the ability to accurately detect, localize, and assess damage in real-time, significantly reducing the risk of catastrophic failure. By leveraging sophisticated algorithms, these systems can analyze data from embedded sensors within the composite materials, identifying anomalies that signify damage. This proactive approach to damage detection allows for timely maintenance and repairs, ensuring the structural integrity of aircraft.
Furthermore, ML-based SHM contributes to the development of predictive maintenance models, enabling aerospace engineers to anticipate potential issues before they escalate into serious problems. This predictive capability not only ensures the safety of the aircraft but also optimizes maintenance schedules, reducing downtime and extending the lifespan of the aerostructure components.
The success of implementing an ML-based SHM system hinges on continuous improvement and adaptation. By feeding new data into the ML models, the system evolves, improving its accuracy and reliability. This dynamic approach to SHM underscores the potential of ML to enhance aerospace safety, offering a forward-thinking solution to the challenges of maintaining composite aerostructures.
We would like to extend my gratitude to I.E. Saramantas, P.E. Spiliotopoulos, F.T. Fera, D.M. Bourdalos, J.S. Sakellariou, S.D. Fassois, Y. Ofir, I. Kressel, M. Tur, and C. Spandonidis for their invaluable contribution to the field of aerospace engineering. Their work on "A vibration-based Machine Learning Structural Health Monitoring methodology for populations of composite aerostructures under uncertainty" has significantly advanced our understanding and capabilities in structural health monitoring, paving the way for safer and more efficient aerospace structures.
Discover the future of composite manufacturing with Addcomposites! Here's how you can get involved:
At Addcomposites, we are dedicated to revolutionizing composite manufacturing. Our AFP systems and comprehensive support services are waiting for you to harness. So, don't wait – get started on your journey to the future of manufacturing today!
Structural Health Monitoring (SHM) in composite aerostructures presents a complex set of challenges, primarily due to the intricate nature of composite materials and the critical demand for maintaining aerospace safety standards. Composites, characterized by their lightweight and high-strength properties, are increasingly used in aerospace applications, leading to a significant need for effective SHM systems that can detect, localize, and characterize damage accurately.
One of the key challenges in SHM is the detection of damage types that are not readily visible or easily identifiable, such as delaminations or micro-cracks within the composite layers. These damage types can compromise the structural integrity of aerostructures but might not be detectable through traditional inspection methods. Furthermore, the heterogeneous composition of composites adds another layer of complexity, as damage propagation behaves differently compared to homogeneous materials.
The blog highlights the importance of developing sophisticated SHM methodologies capable of overcoming these challenges. It emphasizes the need for systems that can operate under the uncertainties inherent in composite materials, such as variations in material properties, environmental conditions, and operational loads. Achieving this requires a multidisciplinary approach, combining knowledge from material science, mechanical engineering, and data analytics.
Advancements in sensor technologies and machine learning algorithms offer promising solutions to these challenges. By integrating sensors within composite structures, real-time data collection becomes feasible, allowing for the continuous monitoring of structural health. Machine learning algorithms can then analyze this data, learning from patterns and anomalies to detect and characterize damage more accurately.
However, the development and implementation of these advanced SHM systems are not without its hurdles. Technical limitations, such as sensor sensitivity, data processing capabilities, and algorithm robustness, need to be addressed. Additionally, there's a need for extensive validation and testing to ensure reliability and effectiveness in real-world applications.
The task of detecting and characterizing damage within composite aerostructures is notably complicated by the presence of uncertainty. This uncertainty stems from several sources, including the variability in material properties, the influence of environmental conditions on the structure, and the complexities associated with the damage itself, such as its location, size, and type. Traditional methods of structural health monitoring (SHM) often struggle to accurately identify damage under these uncertain conditions, leading to the need for more sophisticated approaches.
Machine learning (ML) offers a powerful solution to this challenge, utilizing algorithms capable of learning from data to detect patterns and anomalies indicative of damage. The application of ML in SHM allows for the analysis of vast amounts of data generated by sensors embedded in the composite structures. These algorithms can adapt to the inherent uncertainties, improving their ability to detect and characterize damage over time.
However, the development and deployment of ML-based SHM systems are not without challenges. The accuracy of these systems heavily depends on the quality and quantity of the data available for training the algorithms. Ensuring that the ML models are trained on comprehensive datasets that accurately represent the range of possible damage scenarios is crucial. Additionally, the interpretability of ML algorithms is essential for gaining the trust of engineers and technicians who rely on these systems to make critical decisions regarding the maintenance and safety of aerostructures.
The advent of machine learning (ML) has significantly shifted the paradigm for damage detection in aerospace composites. By harnessing the power of ML algorithms, the process transcends the limitations of traditional methods, offering a dynamic approach capable of adapting to the complexities inherent in composite materials. This section delves into the methodology and implementation of ML for detecting and characterizing damage within composite aerostructures, highlighting its potential to revolutionize structural health monitoring (SHM).
At the core of this approach is the development of sophisticated ML models trained on data collected from embedded sensors within the composite structures. These sensors provide a continuous stream of data, capturing subtle changes in the material's behavior that may indicate the presence of damage. Through advanced data processing and analysis techniques, ML algorithms can identify patterns and anomalies within this data, flagging potential damage sites for further inspection.
The process involves several key steps, starting with data acquisition and preprocessing to ensure that the ML models receive high-quality inputs. Feature extraction is then performed, isolating the most relevant information from the sensor data to improve the efficiency and accuracy of the detection process. With the features identified, the ML models are trained to recognize the characteristics of damage, learning from examples to refine their predictive capabilities.
Innovating aerospace safety through machine learning (ML) in structural health monitoring (SHM) offers a transformative approach to ensuring the integrity and longevity of composite aerostructures. This section discusses the solution's implementation, emphasizing its potential to revolutionize aerospace safety protocols.
The incorporation of ML into SHM systems enhances the ability to accurately detect, localize, and assess damage in real-time, significantly reducing the risk of catastrophic failure. By leveraging sophisticated algorithms, these systems can analyze data from embedded sensors within the composite materials, identifying anomalies that signify damage. This proactive approach to damage detection allows for timely maintenance and repairs, ensuring the structural integrity of aircraft.
Furthermore, ML-based SHM contributes to the development of predictive maintenance models, enabling aerospace engineers to anticipate potential issues before they escalate into serious problems. This predictive capability not only ensures the safety of the aircraft but also optimizes maintenance schedules, reducing downtime and extending the lifespan of the aerostructure components.
The success of implementing an ML-based SHM system hinges on continuous improvement and adaptation. By feeding new data into the ML models, the system evolves, improving its accuracy and reliability. This dynamic approach to SHM underscores the potential of ML to enhance aerospace safety, offering a forward-thinking solution to the challenges of maintaining composite aerostructures.
We would like to extend my gratitude to I.E. Saramantas, P.E. Spiliotopoulos, F.T. Fera, D.M. Bourdalos, J.S. Sakellariou, S.D. Fassois, Y. Ofir, I. Kressel, M. Tur, and C. Spandonidis for their invaluable contribution to the field of aerospace engineering. Their work on "A vibration-based Machine Learning Structural Health Monitoring methodology for populations of composite aerostructures under uncertainty" has significantly advanced our understanding and capabilities in structural health monitoring, paving the way for safer and more efficient aerospace structures.
Discover the future of composite manufacturing with Addcomposites! Here's how you can get involved:
At Addcomposites, we are dedicated to revolutionizing composite manufacturing. Our AFP systems and comprehensive support services are waiting for you to harness. So, don't wait – get started on your journey to the future of manufacturing today!